In the realm of customer relationship management (CRM), operational CRM has been a game-changer for businesses seeking to enhance customer experiences and drive growth. However, an often overshadowed yet equally vital component is analytical CRM. This article delves into the multifaceted world of analytical CRM, highlighting its applications and providing real-world examples.
Understanding Analytical CRM
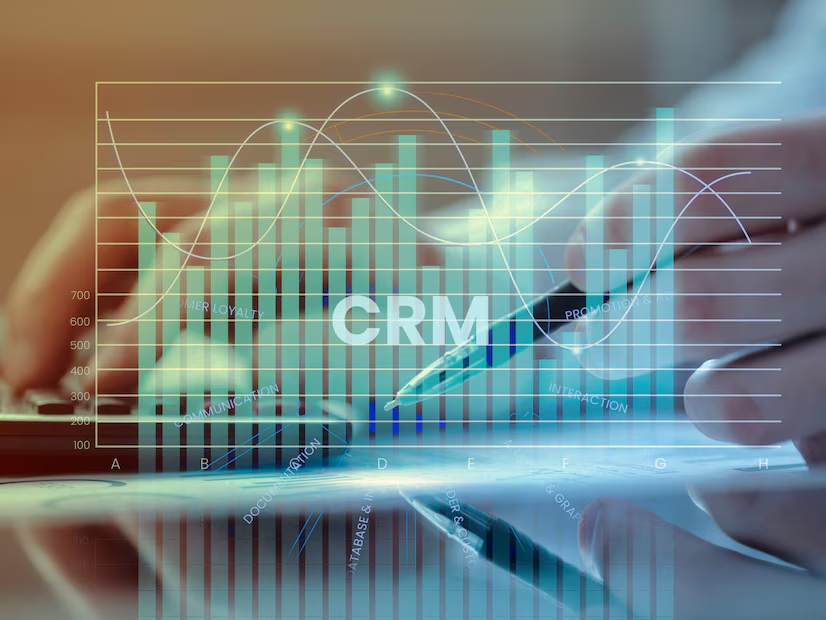
Analytical Customer Relationship Management (CRM) is a vital part of the current approach of businesses that considers the relation between the customer and businesses and maximizes the performance of a business through data analysis. In contrast with operational CRM, which mainly works on the selection of customers and customer interactions, analytical CRM dives into the great source of customer data to form useful insights.
Components of Analytical CRM
In analytical CRM you have several elements that play well together and thus provide you with useful findings. These components include:
Data Warehousing
Data warehousing as the central building block of Analytical CRM is the careful process of assembling, preserving, and organizing large data volumes across many sources. These sources consist of a wide range of documents, which cover transactions, social media interactions, user feedback, web visits, and more. Data warehouses ensure the structuring and indexing of data for their retrieval later on, helping play a crucial role in efficient information analysis. The data warehousing process typically involves:
- Data Collection: Manual data collection from a variety of touchpoints, such as sales, marketing, customer service, and online interactions;
- Data Storage: Keeping the stored data in a centralized repository, mostly designed to provide for logically analytical performance;
- Data Organization: Organizing the info in such a form that the process of substance pull-up and analysis will be easy and efficient.
Data Mining
By using data mining techniques, many interesting patterns, connections and trends correlations will be extracted from the outpoured data repository. These days with the help of multiple algorithms, companies tend to find insights that might be totally missed by conventional analytics. Data mining enables organizations to:
- Identify Purchase Patterns: Discovering a sequence of buying stages and separating one product from the other;
- Detect Anomalies: Distinguishing deviant actions like acts of fraud or users’ negative feedback;
- Customer Segmentation: Stratification of clients by shared features in order to have more effective marketing campaigns.
Predictive Analytics
Predictive analytics algorithms, with their capability of harnessing historical data trends, can forecast the future patterns of customer behavior and market trends. Supplying predictive models helps companies foresee customer needs, allocate resources properly, and customize marketing strategies for the greatest effect. Key applications of predictive analytics include:
- Customer Lifetime Value Prediction: Noting the prospective contribution of patrons in the entire period of their association with the company;
- Churn Prediction: When these regression models figure out the customers at risk of churning, it will facilitate the implementation of proactive retention strategies accordingly;
- Cross-Selling and Upselling: Suggesting items or services users have bought previously to customers.
Segmentation
In segmentation, the customer base is divided into different segments according to the shared sets of characteristics such as demographics, purchasing behavior, and psychographic traits. Through customer segmentation, businesses can indeed adapt their marketing efforts and communication strategies to suit the specific needs and desires of each group. Common segmentation approaches include:
- Demographic Segmentation: Segmentation of customers by age, gender, income, etc;
- Behavioral Segmentation: The consumers are grouped based on their purchase behavior, frequency of interaction, etc;
- Psychographic Segmentation: According to lifestyle, values, and attitudes segmentation should be done.
Benefits of Analytical CRM
Implementing Analytical CRM offers a multitude of benefits to businesses across various industries:
Enhanced Customer Insights
Analytical CRM enables businesses to explore deep into the massive volumes of customer information, giving valuable insights into customer preferences, wants, and practices. Based on the use of cutting-edge analytic tools, businesses can gain a deep understanding of their client base regardless of demographics or purchase behavior among other touch points.
- Customer Segmentation: Through segmentation analysis, companies are able to divide customers into different groups based on similarities in their attributes and behavior. Such segmentation makes marketing more accurate and communication more personalized;
- Predictive Analytics: Using predictive analytics techniques such as machine learning algorithms, business organizations can predict future trends in consumers’ actions. With this knowledge at hand, companies can prepare themselves for better services according to the changing demands by their clients and even nip challenges before they affect many people;
- 360-Degree View of the Customer: Integrating data from multiple sources like sales channels, marketing channels, customer care units, etc., helps firms create a complete picture of each customer. This kind of holistic viewpoint leads to better decision-making by ensuring that interactions with buyers are made at all stages in relation with the customers’ vision journey.
Improved Marketing Effectiveness
Analytical customer relationship management entails companies being able to target demographics with advertising that is, by nature, very relevant to the targeted individuals. This works toward allowing businesses to increase their marketing effectiveness and return on investment (ROI) by developing communications that are personalized and relevant.
- Customized Content: When a company is able to better reach the customers, one such possibility will be to identify the unique styles and behaviors in relation to a series of marketing materials and offers, which have to be tailored to them. The participation of personalization will better help the brand connect with their customers;
- Optimizing the Campaign: The business will continue to strengthen and optimize the marketing strategy in real time, controlling, monitoring, and analyzing campaign performance metrics. It would help an organization identify what works best and the areas it could take an opportunity for improvement so that an organization can optimize marketing, making it efficient and most impactful.
Increased Customer Retention
Analytical CRM allows proactive customer retention by enabling an understanding of at-risk customers and designing interventions to exactly meet the requirement for retention. Building loyalty and churning down is through nurturing relationships with customers by delivering compelling experiences.
- Early Warning Systems: Predictive analytics helps in building early warning systems, flagging any churn potential signals like low engagement or negative sentiment. If they are identified as ‘at-risk,’ the company might take proactive steps in reducing churn and hence save valuable relationships by doing so at a sufficiently early stage;
- Personalized Retention Strategies: The insights around the individual customer’s preferences and issues would mean that the business could be able to personalize the retention strategies to respond to the uniqueness of what each customer would need. Such things as personal offers, proactive support, and loyalty programs point to the organizational level of customer-centricity devotion.
Optimized Cross-Selling and Upselling
This analytic part of CRM easily pinpoints cross-selling and upselling opportunities, looking at customer purchase history, preference, and behavior data. It recommends complementary products or upgraded premiums strategically so that it maximizes customer lifetime value, and with this, a business can drive additional revenues in this way.
- Recommendation Engines: With data-based recommendation engines, companies can suggest shopping to individual customers of products for which he possibly is interested in, based on a history of orders and browsing by the customer. Such precise recommendations are known to improve the shopping experience and have a better shot at succeeding in cross-selling and upselling;
- Behavioral Analysis: Uncovering trends and preferences from the behavioral interaction study of customers and purchase patterns present an opportunity to cross-sell or up-sell. This behavior analysis helps companies in suggesting relevant products or services that will increase the average order value and, therefore, their revenues proactively.
How Analytical CRM Works
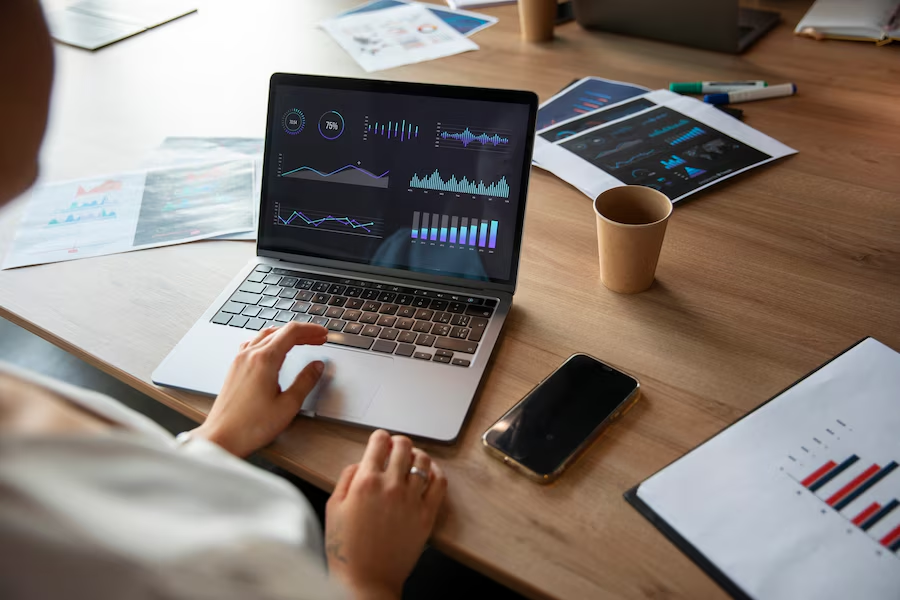
Analytical Customer Relationship Management (CRM) is a modern business strategy tool that gives a company the capability to take advantage of the knowledge extracted from the data for decision-making and interact with customers in a personalized manner. This is how Analytical CRM works:
Data Collection
Analytical CRM begins with the collection of vast amounts of data from diverse sources. These sources include:
- Operational CRM Systems: These are systems designed to capture and store data from customer transactions, which may involve inquiries, purchases, complaints, and feedback;
- Transactional Systems: The sales transactions data, therefore, will emanate from the company’s website interaction data and from the social media data on the various platforms;
- External sources: These may range from market research and demographic data to third-party sources that enhance the dataset with a wider perspective.
Data Processing
Once the raw data is collected, it goes through cleaning, transformation, and integration in order to make it consistent and usable. This includes commonly:
- Data Cleansing: Identifying and rectifying errors, inconsistencies, and duplicates within the dataset to maintain data integrity;
- Data Transformation: Converting data into a standardized format for compatibility and ease of analysis;
- Data Integration: Combining data from various sources into one repository to eliminate silos, which could enable a holistic analysis of the data.
Data Analysis
Analytical CRM employs various analytical techniques to extract meaningful insights from the processed data. Key analytical methods include:
- Descriptive Analytics: Summarizing historical data to understand past trends and patterns, such as customer demographics, purchasing behavior, and preferences;
- Predictive Analytics: Forecasting future outcomes based on historical data and statistical models, enabling proactive decision-making and targeted marketing campaigns;
- Prescriptive Analytics: Recommending optimal actions and strategies by analyzing data patterns and identifying opportunities for improvement, such as personalized product recommendations or churn prevention strategies.
Insight Generation
Through advanced analytics, analytical CRM generates actionable insights that drive strategic decision-making and enhance customer relationships. These insights include:
- Customer Segmentation: Dividing customers into distinct groups based on shared characteristics or behaviors, allowing for targeted marketing and personalized experiences;
- Lifetime Value Analysis: Estimating the potential value of individual customers over their entire relationship with the company, guiding resource allocation and customer prioritization efforts;
- Churn Prediction: Identifying customers at risk of churn based on behavioral indicators, enabling proactive retention initiatives to mitigate attrition.
Application and Optimization
Finally, the insights derived from analytical CRM are applied across various business functions to optimize processes and enhance customer experiences. This involves:
- Marketing Campaign Optimization: Tailoring marketing strategies and messages to specific customer segments, improving campaign effectiveness and ROI;
- Sales Performance Improvement: Providing sales teams with actionable insights and recommendations to prioritize leads, cross-sell/up-sell opportunities, and improve conversion rates;
- Service Enhancement: Personalizing customer interactions and support experiences based on individual preferences and needs, fostering loyalty and satisfaction.
Analytical CRM in Marketing
Let’s delve into the detailed workings of analytical CRM within the realm of marketing:
Customer Segmentation
Analytical CRM facilitates the segmentation of customers into distinct groups based on various criteria such as demographics, purchasing behavior, and preferences. This segmentation allows marketers to tailor their strategies and messages to specific audience segments, maximizing relevance and engagement. The process of customer segmentation involves:
- Demographic Segmentation: Classifying customers based on demographic attributes like age, gender, income level, and geographic location;
- Behavioral Segmentation: Grouping customers according to their purchasing behavior, frequency of purchases, product preferences, and interaction history;
- Psychographic Segmentation: Segmenting customers based on lifestyle, personality traits, values, and interests.
Targeted Messaging
Armed with segmented customer data, marketers can craft personalized and targeted messages that resonate with each audience segment. Analytical CRM enables the customization of marketing communication by:
- Content Personalization: Tailoring marketing messages, offers, and content to match the preferences and needs of specific customer segments;
- Channel Optimization: Identifying the most effective communication channels for reaching each segment, whether it’s email, social media, mobile apps, or direct mail;
- Timing Optimization: Determining the optimal timing for delivering messages based on customer behavior and preferences, maximizing engagement and response rates.
Campaign Effectiveness Measurement
Analytical CRM provides robust tools for measuring the effectiveness of marketing campaigns and analyzing customer responses. By tracking key performance indicators (KPIs) and analyzing campaign metrics, marketers can assess the impact of their initiatives and make data-driven decisions. Key aspects of campaign effectiveness measurement include:
- Conversion Rate Analysis: Evaluating the percentage of recipients who respond to a campaign by taking the desired action, such as making a purchase or signing up for a newsletter;
- ROI Calculation: Calculating the return on investment (ROI) for marketing campaigns by comparing the cost of the campaign to the revenue generated or other predefined metrics;
- Customer Feedback Analysis: Gathering and analyzing customer feedback and sentiment data to understand the effectiveness of messaging and identify areas for improvement.
Refinement of Marketing Strategies
Analyzing customer responses and campaign performance data allows companies to iterate and refine their marketing strategies continuously. By leveraging insights gleaned from analytical CRM, marketers can optimize their tactics and enhance future campaign effectiveness. This iterative process involves:
- A/B Testing: Experimenting with different variations of messaging, offers, and campaign elements to identify the most effective approach;
- Segmentation Refinement: Fine-tuning customer segmentation criteria based on the analysis of campaign outcomes and customer behavior;
- Predictive Modeling: Using historical campaign data to develop predictive models that forecast future customer behavior and inform strategic decision-making.
Sales Optimization Through Analytical CRM
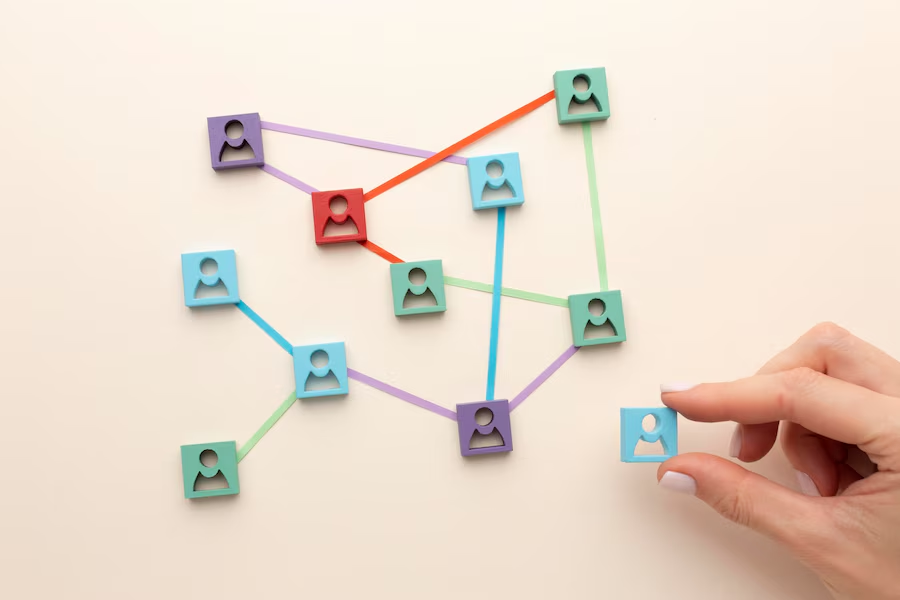
Sales teams use analytical CRM to identify potential leads, assess customer value, and predict future purchasing patterns. This targeted approach ensures that sales efforts are focused on the most promising prospects. Let’s delve into the detailed workings of how analytical CRM enhances sales optimization:
Lead Identification
Analytical CRM enables sales teams to identify potential leads by analyzing various data sources and criteria. By leveraging demographic information, purchasing behavior, and interaction history, sales professionals can pinpoint prospects with the highest likelihood of conversion. Key aspects of lead identification include:
- Data Analysis: Analyzing customer databases, website interactions, social media engagement, and other sources to identify individuals or organizations showing interest in products or services;
- Lead Scoring: Assigning scores or rankings to leads based on predefined criteria such as demographics, engagement level, and purchase intent, prioritizing efforts on leads with the highest potential value;
- Behavioral Analysis: Examining customer behavior and online activities to identify signals of interest or intent, such as repeated visits to product pages or engagement with marketing content.
Customer Value Assessment
Analytical CRM facilitates the assessment of customer value, allowing sales teams to prioritize high-value customers and allocate resources accordingly. By analyzing factors such as lifetime value, purchase history, and revenue potential, sales professionals can focus their efforts on maximizing returns. Key components of customer value assessment include:
- Lifetime Value Analysis: Estimating the potential long-term value of individual customers by considering factors such as past purchases, frequency of interactions, and future revenue potential;
- Segmentation by Value: Segmenting customers into tiers or categories based on their value to the company, allowing sales teams to tailor strategies and engagement approaches accordingly;
- Cross-Selling and Up-Selling Opportunities: Identifying opportunities to sell additional products or services to existing customers based on their past purchasing behavior and preferences, maximizing revenue per customer.
Predictive Analytics for Purchasing Patterns
Analytical CRM leverages predictive analytics to forecast future purchasing patterns and trends, enabling sales teams to anticipate customer needs and proactively address them. By analyzing historical data and employing predictive models, sales professionals can make informed decisions and stay ahead of market dynamics. Key aspects of predictive analytics for purchasing patterns include:
- Forecasting Models: Developing predictive models that anticipate changes in customer behavior and market demand, enabling proactive sales strategies and resource allocation;
- Demand Planning: Anticipating fluctuations in product demand and adjusting inventory levels and production schedules accordingly, ensuring timely availability to meet customer needs;
- Churn Prediction: Identifying customers at risk of churn based on behavioral indicators and implementing retention strategies to mitigate attrition and preserve revenue.
Targeted Sales Efforts
Armed with insights from analytical CRM, sales teams can tailor their efforts and resources toward the most promising prospects and opportunities. By focusing on high-potential leads and personalized engagement strategies, sales professionals can optimize conversion rates and drive revenue growth. Key elements of targeted sales efforts include:
- Personalized Outreach: Crafting personalized sales pitches and messages tailored to the needs and preferences of individual leads, increasing relevance and effectiveness;
- Sales Pipeline Management: Managing the sales pipeline more effectively by prioritizing leads and opportunities based on their likelihood of conversion and potential value;
- Performance Tracking: Monitoring and analyzing sales performance metrics to identify areas for improvement and optimize sales processes and strategies.
Enhancing Customer Service with Analytical CRM
Customer service departments leverage analytical CRM to better understand customer issues and anticipate needs. By analyzing past interactions and feedback, companies can proactively address potential problems, improving overall customer satisfaction. Here’s an in-depth exploration of how analytical CRM enhances customer service:
Understanding Customer Issues
Analytical CRM enables customer service departments to gain a deep understanding of customer issues by analyzing a wide range of data sources, including:
- Interaction History: Analyzing past interactions between customers and the company across various channels such as phone calls, emails, live chat, and social media;
- Feedback and Surveys: Mining customer feedback from surveys, reviews, and social media platforms to identify recurring issues, pain points, and areas for improvement;
- Transactional Data: Examining transactional data to understand purchasing patterns, product usage, and billing inquiries.
Anticipating Needs
By leveraging predictive analytics, analytical CRM empowers customer service teams to anticipate customer needs and proactively address potential problems. Key aspects of anticipating customer needs include:
- Predictive Modeling: Developing models that forecast future customer behavior and trends based on historical data, enabling proactive service strategies;
- Churn Prediction: Identifying customers at risk of churn by analyzing behavioral indicators and implementing retention initiatives to mitigate attrition;
- Issue Resolution Prediction: Anticipating potential service issues or complaints based on historical patterns and taking preemptive action to resolve them before they escalate.
Proactive Problem Resolution
Analytical CRM enables companies to proactively address customer issues and concerns before they become significant problems, thereby improving overall customer satisfaction. This proactive approach involves:
- Real-time Monitoring: Implementing systems to monitor customer feedback and sentiment in real-time, allowing for prompt intervention and resolution;
- Automated Alerts: Setting up automated alerts and notifications to flag potential issues or anomalies based on predefined criteria, enabling swift action by customer service teams;
- Personalized Responses: Tailoring responses and solutions to individual customer needs and preferences, based on insights derived from analytical CRM.
Continuous Improvement
Analytical CRM facilitates continuous improvement in customer service by providing actionable insights and performance metrics to track progress and identify areas for enhancement. Key aspects of continuous improvement include:
- Performance Analytics: Analyzing key performance indicators (KPIs) such as response times, resolution rates, and customer satisfaction scores to measure service effectiveness;
- Root Cause Analysis: Investigating the underlying causes of recurring issues or customer complaints to implement preventive measures and process improvements;
- Feedback Loop Closure: Closing the feedback loop by incorporating customer feedback into product development, service delivery, and process optimization initiatives.
How to Choose the Right Analytical CRM Application
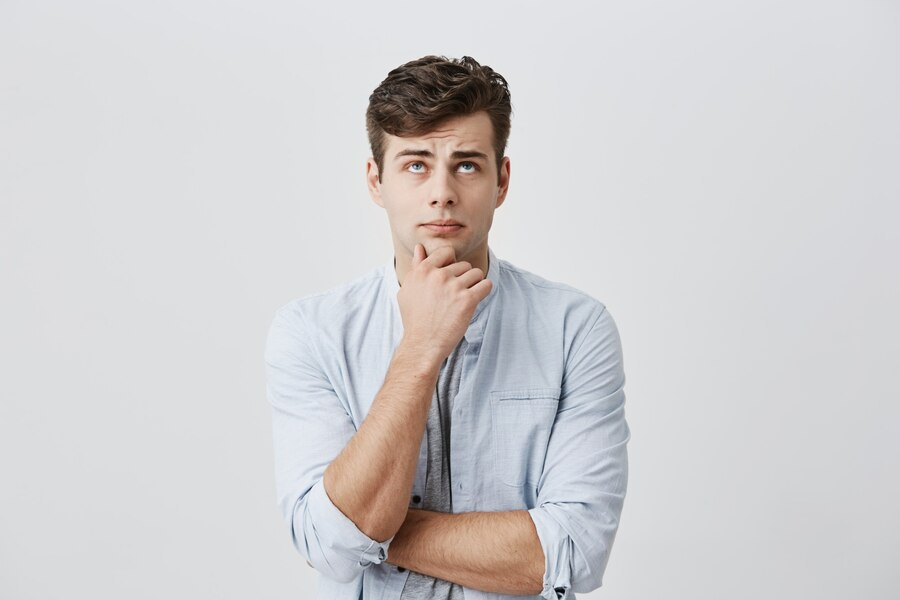
Selecting the right analytical Customer Relationship Management (CRM) application is crucial for maximizing the effectiveness of your data analysis efforts and optimizing business outcomes. Here’s a detailed guide on key factors to consider when choosing an analytical CRM application:
Compatibility with Existing Systems
Ensure that the analytical CRM application seamlessly integrates with your existing systems and infrastructure. Compatibility is essential for smooth data flow and interoperability across different platforms. Consider the following aspects:
- Integration Capabilities: Evaluate whether the CRM application can integrate with your current customer data sources, such as operational CRM systems, transactional databases, and marketing automation tools;
- API Support: Check if the application offers robust APIs (Application Programming Interfaces) for easy integration with third-party applications and custom development solutions;
- Data Formats and Standards: Verify that the CRM application supports common data formats and standards used in your organization, ensuring compatibility and ease of data exchange.
Scalability as Your Business Grows
Choose an analytical CRM application that can scale alongside your business as it grows and evolves. Scalability is essential for accommodating increasing data volumes, user counts, and business complexity. Consider the following scalability factors:
- Performance: Assess the application’s performance under varying workloads and data volumes to ensure it can handle growing demands without compromising speed or efficiency;
- Storage and Processing Capacity: Evaluate the scalability of the CRM application’s storage and processing capabilities to accommodate expanding data requirements over time;
- User Licensing and Pricing: Ensure that the application’s licensing model and pricing structure are flexible enough to scale with your business needs without incurring prohibitive costs.
Range of Features Offered
Evaluate the breadth and depth of features offered by the analytical CRM application to ensure it meets your specific analytical and business requirements. Look for comprehensive functionality that covers key aspects of data analysis, reporting, and decision support. Consider the following feature considerations:
- Data Analytics Tools: Assess the application’s capabilities for data exploration, visualization, and advanced analytics, including predictive modeling, segmentation, and trend analysis;
- Reporting and Dashboarding: Look for robust reporting and dashboarding features that provide actionable insights and facilitate informed decision-making for sales, marketing, and customer service;
- Customization and Extensibility: Determine whether the application allows for customization and extensibility to tailor functionality to your unique business processes and analytical needs.
User-Friendliness and Support Services
Choose an analytical CRM application that is user-friendly and supported by comprehensive training and support services to ensure successful adoption and ongoing usage. Consider the following usability and support factors:
- Ease of Use: Evaluate the application’s user interface and workflow to ensure it is intuitive and accessible for users with varying levels of technical expertise;
- Training and Documentation: Look for vendor-provided training resources, documentation, and user guides to facilitate onboarding and skill development for users;
- Customer Support: Assess the quality and responsiveness of the vendor’s customer support services, including technical support, troubleshooting, and issue resolution.
Conclusion
Analytical CRM applications are invaluable in today’s data-driven business environment. They enhance understanding of customer behavior, improve decision-making, and ultimately contribute to a more personalized customer experience. Businesses leveraging what is analytical CRM and embracing its examples are well-positioned to thrive in the competitive market.
FAQ
While operational CRM focuses on managing customer interactions, analytical CRM is about analyzing customer data to drive better business decisions.
Absolutely. Analytical CRM applications can scale to fit the needs of businesses of all sizes, offering insights to drive growth and improve customer engagement.
Costs vary depending on the scale and complexity of the system. However, the return on investment can be significant in terms of improved customer insights and business efficiency.